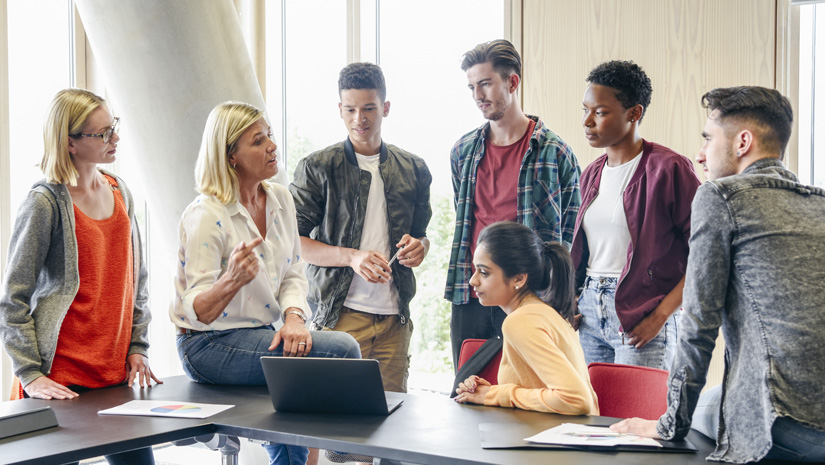
Key insights
- Declining enrollment is a concern for many higher education institutions, but getting the numbers to move in the right direction may take more than bringing in new students.
- The first step is understanding the challenge — and that can mean digging into data in a new way.
- Machine learning can help uncover data trends and opportunities, so your organization can create tactics to address issues within targeted student populations.
Ready to uncover new insight from your data?
A falling birthrate, changing social norms, a long-lived pandemic, and a multitude of other factors contribute to an unfortunate truth: in the next four to five years, higher education enrollment is likely to decline for many institutions.
This puts an already overworked section of college administrators — those in charge of enrollment and recruiting — in a difficult position. How to recruit even more students from a smaller and smaller pool? While there are many smart and hardworking folks tackling this problem, looking at it only from a recruitment perspective may be too shortsighted. What do we mean by that?
Step back to analyze the challenge
We see many schools trying to recruit themselves out of a retention problem.
Based on a sampling of publicly available data, a retention rate of 40 – 70% is not uncommon for many schools. That means that for every 10 students the enrollment department registers, as many as six of those won’t stay the full four years.
We’ve worked with some schools that found a 0.5% increase in retention translates to $200,000 in opportunity costs saved.
And with the enrollment cliff looming, those attrition numbers are only going to continue to increase.
So what do we recommend? A new retention concept.
Identify the data and variables that matter
Retention professionals (i.e., student success, specific “retention taskforces,” etc.) are often having to do more with less. They also have myriad data at their fingertips but aren’t sure where to start. What should they focus on?
- GPA?
- Qualitative advisor updates?
- Students not in sports?
- Legacy students?
The list goes on.
When a school is unable to prioritize, it may institute “blanket solutions” that cater to everyone — even those students who were low risk of attrition in the first place.
Maybe every freshman gets a “freshman mentor” or the administration requires advisors to meet with every student a set number of times per year and escalate any concerns. While not bad ideas, the main issue still exists: Blanket approaches don’t allow you to target specific “at-risk populations.” The low-attrition risk group is unchanged, and the high-attrition risk group still doesn’t get enough support.
There must be a better way. Enter machine learning.
Machine learning: a case study
Understanding the situation
A private nonprofit liberal arts school in the Midwest wanted to see if there was a way to use machine learning and data to get a handle on retention.
This school had tenured, brilliant folks in the administration, and administrators had a “gut feel” about the things that might contribute to potential retention challenges. But they were bumping up against limited time, money, and energy — and a lack of knowledge about which data points mattered.
Exploring the challenge
The CLA team started with the high-level question:
Can we build a machine learning model, train it on historical data, and use it to find the top five most important variables related to retention?
The answer was yes.
Knowing we could use machine learning to generally find relationships within a disparate list of variables, the school asked us to look at things like:
- On-campus versus off-campus students
- Distance
- Career GPA
- Billing cohort (i.e., their year in school)
- Major
- Advisor
- Sports
- Club participation
- 15 other similar variables
Our data science and machine learning team set to work to turn this business intelligence into action.
Achieving results
CLA’s Early Warning Indicator Tool is designed to uncover the most important variables related to retention. Its machine learning capabilities help us focus on those critical few factors that are highly correlated to attrition and retention while ignoring the trivial many factors that don’t matter.
This Midwestern school found the factors below were most likely to impact retention:
- Distance — How far did the student live from campus?
- Discount rate — After all tuition assistance, relatively how much tuition did this student pay?
- Hours enrolled — Massive retention boost if students were above 15 hours
- Career GPA — Lower GPA students were at higher risk of attrition
- Building code — What dorm? On campus versus off campus
If we use “hours enrolled” as an example, we start to see the type of data the model can provide. The model split hours enrolled into three cohorts, each with markedly different retention numbers:
- Less than full time (fewer than 13 credit hours) — Extremely high risk of attrition
- Full time (13 – 15 credit hours) — Moderately high risk of attrition
- Full capacity (greater than 15 credit hours) — Low risk of attrition
For administrators, the implications were clear. Instead of a blanket approach, they now had a specific subset of students to which they could dedicate specific resources: the highest-risk population.
This principle repeated itself in all five variables above, giving the administration specific subsets of students that they can directly influence.
Explainable AI plots
One typical critique of machine learning models is they tend to be a “black box.” If the model recommends something, the user is supposed to trust that because it is the model it makes sense. This leads to a lack of trust and understanding of the tool.
To address this concern, we built “explainable AI plots” into our model. Below, these plots explain, at the student level, why the model predicted what it did. Briefly, it shows the model predicts 84% chance this student will attrit. Why?
The green lines represent risk factors associated with the prediction of “this student will leave.” Factors including hours enrolled, what building they were in, what year student they were, and their academic status — which all contributed to this prediction. We found that hours enrolled and building code had the largest impact.
Each student has an explainable AI plot that gives further information on why the model predicted what it did.
How we can help
In today’s environment of declining enrollment numbers, higher education institution must be proactive instead of reactive. You need to be armed with surgical approaches instead of blanket ones. But to do so successfully, you need to understand what factors influence retention and what factors don’t.
CLA’s data analytics and insight team can help your organization dive deeper into the data that can help future proof your retention process and offset the impact of the impending enrollment cliff. Fill out the form below to learn more.