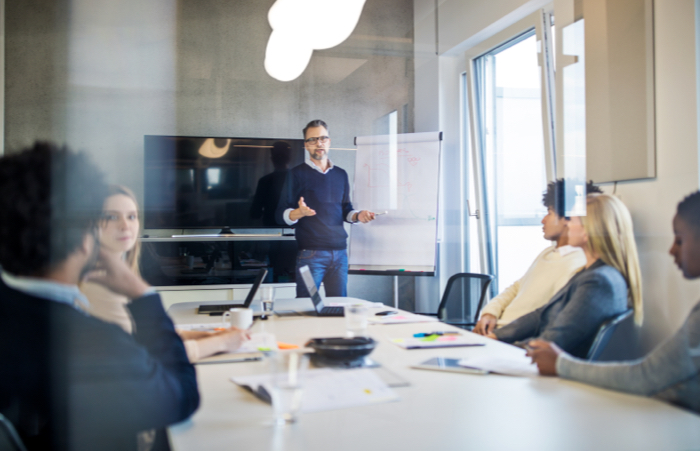
Predictive analytics can reveal the hidden potential in your data. Make real-time decisions that can increase efficiency and help your organization create a strategic, data-driven approach.
Key insights
- Predictive analytics is a form of machine learning that uses historical data to make likely predictions about future outcomes.
- When it comes to meeting tight deadlines and customer delivery demands, predictive maintenance can be a valuable tool.
- The quality of data is essential; it must understood and implemented effectively.
Want to put predictive analytics to work for you?
As data becomes an increasingly important asset of modern manufacturing businesses, manufacturers are increasingly relying on predictive analytics to better achieve their goals and deliver for customers.
Simply put, predictive analytics is a form of machine learning that uses historical data to make predictions about future outcomes. Think for a moment about the sheer volume of data a manufacturing business manages — from specific measurements to power and water consumption to maintenance requirements and delivery schedules. Through predictive analytics, calculations can be made to help organizations achieve a positive outcome, and to understand more about operational data so they can learn and improve.
Predictive analytics can be particularly valuable in the area of predictive maintenance, which uses compiled sensor data to determine when certain machines need repairs. Few things are more disruptive to a manufacturing business than unexpected delays caused by problems with machinery. With predictive maintenance, this might be avoided — data is compiled and can be used to determine when certain machines need to be taken offline for repairs or upgrades. When it comes to meeting tight deadlines and customer delivery demands, this is a much-preferred system to simply hoping a machine doesn’t break down before its usual maintenance date.
A major reward of the predictive analytics process, particularly in the manufacturing sector when timely and uninterrupted production and delivery is so essential, is that it can create a better understanding of your data. Predictive models can shorten the time between taking a custom product order and customer delivery. The predictive analytics model allows the manufacturer to plug in parameters and receive a prediction of the likely outcome — and results can be seen in minutes rather than hours.
While predictive analytics is increasingly beneficial, it does come with its challenges. If the right kind of data is not collected, it makes creating a model difficult. This can be addressed with a more robust data collection and quality assurance process, which could offer a better glimpse as to why a product is or isn’t working. Predictive analytics depends on all relevant data being accessible and brought together into one place to train a predictive model. In some instances, equipment upgrades — especially machines with sensors more conducive to a predictive analytics setting — could also help.
One consideration that’s not always apparent: the granularity of data being measured affects what kinds of predictions are possible. For example, what if a manufacturer has orders for a custom product that only come in once or twice a month? Taking a daily look at something that happens much less frequently can be inefficient and oftentimes misleading. In this example, changing the level of granularity for predictions to a monthly view will reduce some of the unnecessarily “noisy” ambiguity around whether an order might come in on a Friday at the beginning of the month versus a Tuesday in the middle.
The key to making predictive analytics work is the quality and quantity of the existing historical data. With enough precise data that identify how inputs to a process are related to the outputs, a predictive model can be used to an organization’s advantage. If these criteria are met, the investment of time and resources can help decrease downtime, reduce costs and waste, and identify necessary interventions before they become a problem. Predictive analytics is an increasingly important part of the manufacturing toolkit for companies that want to make strategic decisions with real-time data.
How we can help
CLA manufacturing professionals keep a close watch over current issues and trends. Our deep industry knowledge and experience can provide valuable resources, and our data analytics professionals can guide you every step of the way in developing your data analysis approach.